Introduction to Research Design and Statistics
The Logic of Hypothesis Testing
Hypotheses
We will be discussing two kinds of hypotheses, research hypotheses and statistical
hypotheses. Research hypotheses are the ones that are stated in relatively plain English about
what you think will be the outcome of the research.
Examples:
- Consumption of sugar makes children more active or hyperactive.
- Phonics is better for teaching reading than is whole language.
These are
statements concerning expected outcomes.
Statistical hypotheses are probabilistic mathematical statements concerning population values,
stated in terms of the parameters used in the research.
Statistical Hypotheses
There are two types of statistical hypotheses, null hypotheses and alternative hypotheses. Null
hypotheses are denoted H0: while alternative hypotheses can be denoted as either
H1: or Ha:.
Examples:
H0: μ1 = μ2
H1: μ1 ≠ μ2
H0: μi = μj for all i and j
H1: μi ≠ μj for some i and j
Types of Errors
| | Truth about Population |
| | H0 True | H0 False
|
---|
Decision Based on Sample | Reject H0 | Type I Error | Correct Decision |
Fail To Reject H0 | Correct Decision | Type II Error |
Probabilities
α is the probability of making a Type I Error and is called
the level of significance or the α-level.
α is the probability that you will reject the null hypothesis
when it is true.
1 - α is called the level of confidence.
β is the probability of making a Type II Error.
1 - β is known as the power of a test.
The power of a test is the ability of a statistical test to detect true effects when they exist.
Thus, power is the probability that you will reject the null hypothesis when it is false, i.e.,
the probability that you will detect true differences when they exist.
Researchers can select the alpha level they wish to use. Common alpha levels include .05 and .01.
Beta and power are controlled indirectly
through
- Sample Size
- Alpha Level
- Strength of the Treatment/Effect Size
- Amount of Variability (in paticular error variability)
Choosing an Alpha Level
Make α too large and you will commit too many Type I Errors.
Make α too small and you will not have enough power to detect true effects when they exist.
Abuses of Statistical Tests
Statistical inference is not valid for all sets of data.
Beware of searching for significance (kitchen sink research).
Don't overlook non-significance.
The Meaning of Statistical Significance
It does not mean that the effect is large, important or meaningful.
The observed result is unlikely to occurr by chance alone.
It means that the observed effects are unlikely due to chance.
It means that the results are reliable and likely to be repeatable.
The P-Value
The probability, computed assuming that H0 is true.
The smaller the P-value the more likely that we will reject H0.
Probability Regions
Rejection regions.
Failure to reject region.
One-tail vs Two-tail Tests
One-tail test have a single rejection
Two-tail tests have two rejection regions.
H0: μ1 = μ2
H1: μ1 ≠ μ2
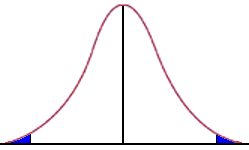
Distributions based upon squared values, such as, chi-square and F, have all of the
rejection region in one tail but are, in fact, two tail tests of hypotheses.
Critical Values
Critical values of a statistic indicate the beginning of the rejection regions. For example,
consider some criticla values for the standard normal distribution:
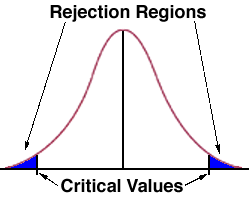
| | One-tail | Two-tail |
Alpha | .01 | 2.33 | ±2.58 |
.05 | 1.645 | ±1.96 |
Intro Home Page
Phil Ender, 30Jun98